Established as a Z_GIS research area in 2021, our research in EO Analytics focuses on multi-scale and multi-purpose big EO data applications and their challenges, focusing primarily on free and open data archives (e.g. Copernicus) and integrating geographic information and objects into big EO data analytics. We aim towards automated semantic image understanding, combined spatial and temporal analysis in large imagery databases, and improved exploration and understanding of uncertainty and inhomogeneity in coverage and quality inherent to global EO archives and analysis. Combining advances in the fields of geography, image understanding, remote sensing, image processing, computer vision, spatial analysis and computer science, we endeavour to develop a collection of adaptive, interdisciplinary solutions necessary to approach challenges posed by big EO data from different angles and application perspectives.
Find us on LinkedIn: LinkedIn: https://www.linkedin.com/company/eo-analytics-lab
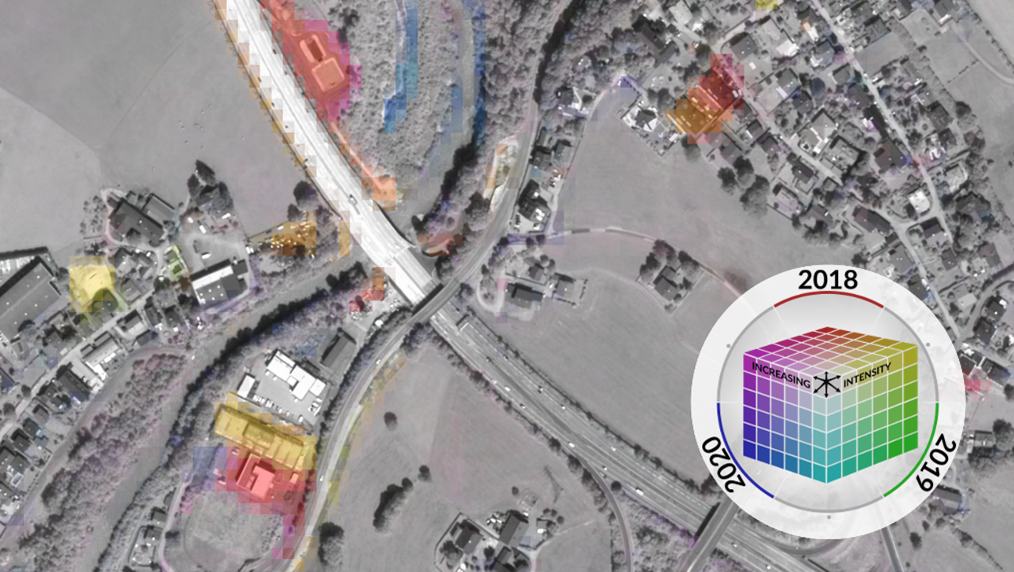
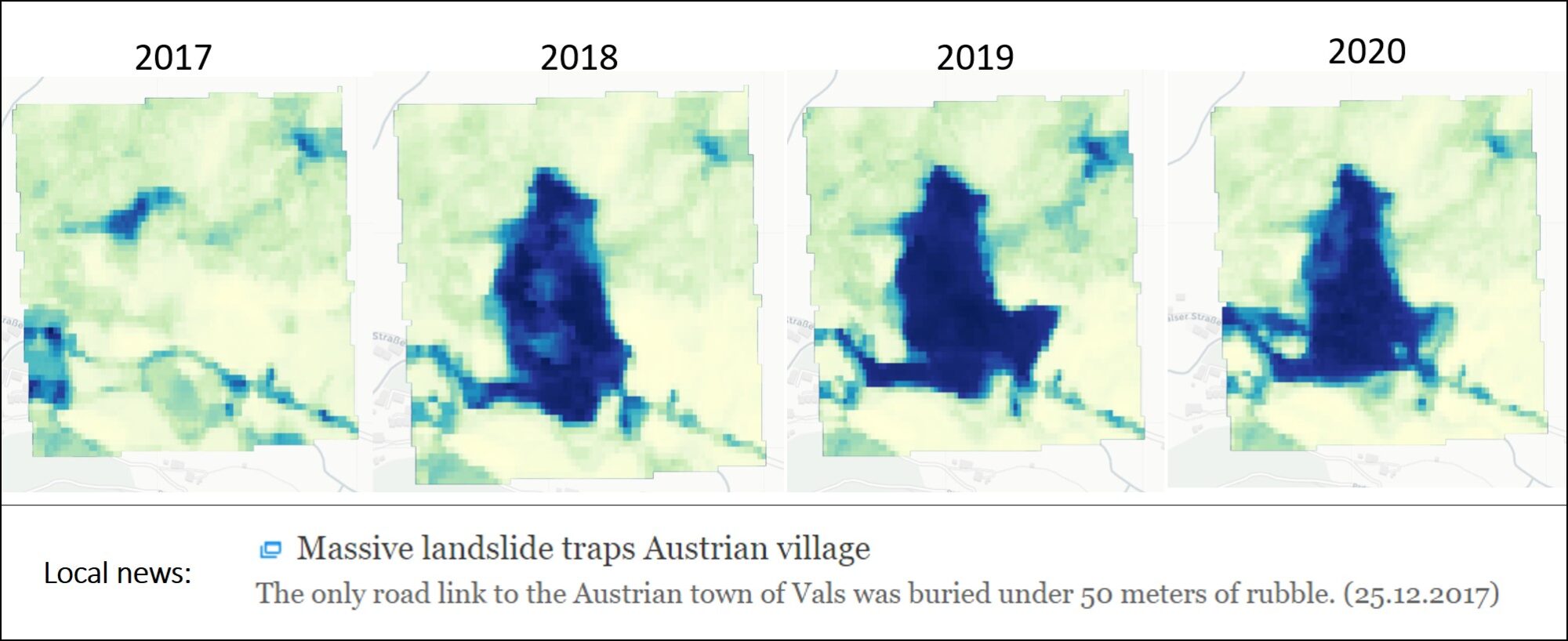